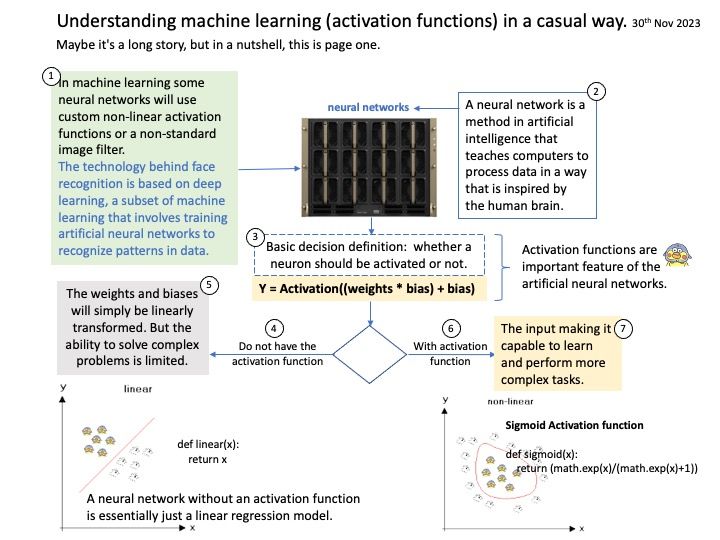
Preface: Maybe it’s a long story, but in a nutshell, this is page one. In fact, when you start studying on your first day. No matter it is an overview of AI technology. The information covers advanced mathematics, graphics and technical terminology. It will reduce your interest. In fact, the world of mathematics is complex. If a child is naturally insensitive to mathematical calculations. Could it be said that he is not suitable for working in artificial intelligence technology? The answer is not absolute. For example: Computer assembly language is difficult and complex to remember. Therefore, the solution is to develop other programming languages and then convert (compile) them into machine language. This is a successful outcome in today’s technological world. Therefore, many people believe that artificial intelligence technology should help humans in other ways rather than replace human work.
Background: The machine learning process requires CPUs and GPUs. GPUs are used to train large deep learning models, while CPUs are good for data preparation, feature extraction, and small-scale models. For inference and hyperparameter tweaking, CPUs and GPUs may both be utilized.
CPU and GPU memory coherence requires data transfer, and requires defining what areas of memory are shared and with which GPUs.
Long story short: Cognition refers to the process of acquiring knowledge and understanding through thinking, experience and senses. In machine learning some neural networks will use custom non-linear activation functions or a non-standard image filter.
The technology behind facial recognition is based on deep learning, a subset of machine learning that involves training artificial neural networks to recognize patterns in data.
Ref: Non-Linear Activation Functions. The non-linear functions are known to be the most used activation functions. It makes it easy for a neural network model to adapt with a variety of data. Adaptive neural networks have the ability to overcome some significant challenges faced by artificial neural networks. The adaptability reduces the time required to train neural networks and also makes a neural model scalable as they can adapt to structure and input data at any point in time while training.