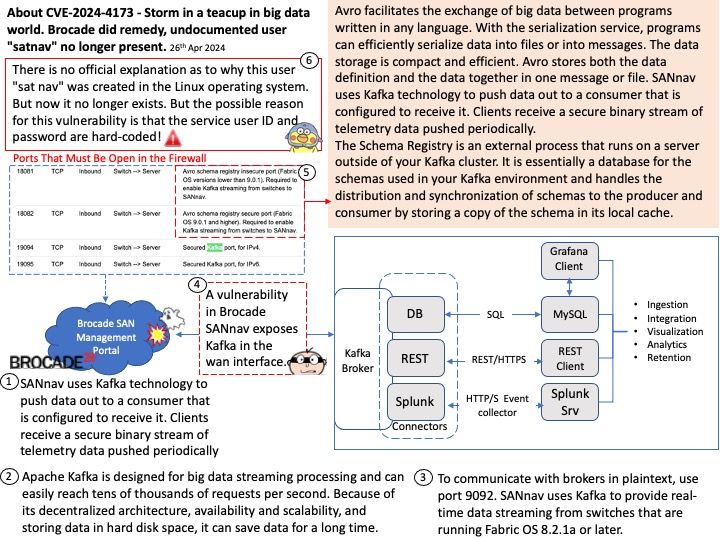
Preface: Apache Kafka was originally developed by Linkedin to solve data pipeline problems, and was later donated to the Apache Foundation as an open source project.
Background: Avro facilitates the exchange of big data between programs written in any language. With the serialization service, programs can efficiently serialize data into files or into messages. The data storage is compact and efficient. Avro stores both the data definition and the data together in one message or file. SANnav uses Kafka technology to push data out to a consumer that is configured to receive it. Clients receive a secure binary stream of telemetry data pushed periodically.
The Schema Registry is an external process that runs on a server outside of your Kafka cluster. It is essentially a database for the schemas used in your Kafka environment and handles the distribution and synchronization of schemas to the producer and consumer by storing a copy of the schema in its local cache.
Vulnerability details: A vulnerability in Brocade SANnav exposes Kafka in the wan interface. The vulnerability could allow an unauthenticated attacker to perform various attacks, including DOS against the Brocade SANnav.
Remark: There is no official explanation as to why this user “sat nav” was created in the Linux operating system. But now it no longer exists. But the possible reason for this vulnerability is that the service user ID and password are hard-coded!
Official announcement: Please refer to the link for details – https://nvd.nist.gov/vuln/detail/CVE-2024-4173